How AI Can Erode Trust

IF you spend enough time in the artificial intelligence (AI) ethics space, you’ll likely come across calls for ‘transparent’ AI. If you haven’t, see, for example, this great piece on transparency and Large Language Models. The need for transparency, in the commonly adopted meaning, is often invoked in discussions emphasising the need for interpretable autonomous decision procedures – to avoid the so-called black box problem.
[T]he black box problem stems from the difficulty in understanding how AI systems and machine learning models process data and generate predictions or decisions. These models often rely on intricate algorithms that are not easily understandable to humans, leading to a lack of accountability and trust.
Undoubtedly, the transparency of AI systems is important. From text to images to recommendations and suggestions, AI systems can generate vast amounts of varied output. Being unable to pinpoint how these outputs are reached not only complicates matters of responsibility and accountability but also worries those with more existential concerns about being able to ‘control’ AI systems.
A less common, but perhaps just as important, meaning of transparent AI refers to the transparent use of AI.
The advent of publicly accessible generative AI – like ChatGPT and text-to-image generators like MidJourney – has already set off alarm bells to this effect. Worry about deepfakes is prevalent (here’s an example of its political implications) and in the education sector, there is rife concern about students committing ‘AI plagiarism’. In response, plagiarism-detection software like TurnItIn introduced AI detection measures. Whilst these don’t work very well, Adobe launched the much more promising Content Authenticity Initiative.
Here, I want to dig into the root of why AI disclosure seems important. In the cases referred to above – deepfakes and AI plagiarism – whilst AI non-disclosure enables their badness, there are external reasons why AI transparency is important.
Students passing off another’s work as their own . . .
Users generating videos of people doing things they’ve never done, in locations they’ve never been, saying things they’ve never said . . .
These are bad, but not just because it wasn’t disclosed that they were achieved through AI.
Plainly, my answer is that AI non-disclosure is deceptive. To understand the real reason why AI disclosure is important, we have to focus on how AI deception preys on and erodes our sense of trust, only to supplant it with a sense of distrust.
(Caveat: I don’t want to go into exactly how we ought to define deception, so I’ll stipulate here that someone deceives if they intentionally make a known falsity appear true to another person.)
Trust: When AI Creates, Do We Value It Less?
First, consider whether and why non-AI disclosure could be intentional. Why would we choose not to disclose the use of AI?
A good place to start answering this question is to ask why we think it matters in the first place. Why does it matter whether the picture I’m looking at, the blog post I’m reading, or the podcast I’m listening to, has been produced by an AI? Consider the following:
Imagine you are presented with two pieces of art. They’re both strikingly beautiful, instilling in you a feeling of euphoria and awe. Much to your dismay, however, you are only allowed one. Finally, after much deliberation, you settle on option A. However, just as you go to shake hands with the art dealer, they inform you that option A was, in fact, created by AI. Does this fact make you rethink your decision?
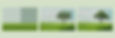
Intuitions may vary, but I take it that for many it would. It seems to matter that this blogpost was written by me, a human, and not an AI. If you’re still not convinced, this phenomenon has been found to be the case. Moffat and Kelly (2006), for example, reported that people tended to dislike computer-generated music just because it was computer-generated; meanwhile, Chamberlain and colleagues (2018) concluded that having knowledge “an artwork is computer-generated impacts negatively upon aesthetic ratings” (p.188).
Call this computational bias (CB):
humans are predisposed to prefer human works over computational ones.
Why?
Well, concerning art, it might be to do with a “lack of humanness and mind” or, simply, because art is additionally evaluated by the effort gone into it and computer-generated art is not as labour-intensive as human art (Lima et.al 2021, p.10).
One candidate inapplicable to art, but highly relevant to text, is trust. It is quite common to hear about generative systems like Chat-GPT getting things wrong. Not only do they seem to make things up, they confidently make frequent mistakes, and are incredibly fickle when challenged. If we know a blog post has been written by an AI we will be less likely to trust (and, therefore, value) what it says.
CB highlights how who (or what) produces an output affects how we subsequently evaluate it. It is this bias that I think underlies the importance of disclosing the use of AI: CB provides a reason not to.
There is reason to intentionally hide the use of AI: once we know, we value it less.
Distrust: AI Sowing the Seeds
Second, for AI non-disclosure to be deceptive, we have to start off assuming a particular output was human-generated, rather than AI-generated.
Reflect on the last corporate blogpost, email newsletter, website you read; video you watched; or song you listened to.
Now ask yourself: was your default assumption that it was produced by a human, or an AI?
For most, I think the current default assumption is that what they read is written by a human. If bad actors wish to be deceptive, this is the assumption they will play off.

Imagine, for instance, that the art dealer did not disclose to you the artwork you chose was AI-generated. Perhaps you would never find out, and it’d be happy days, because in the absence of being told otherwise you had assumed it was human-generated. But even so, or let's say you did, you would feel as though you made the wrong choice and would thus feel as though you had been deceived.
In itself, deception is wrong. But it might also become symptomatic of something worse:
It’s conceivable that as generative AI becomes even more publicly accessible, this default assumption will become jaded.
No longer will we assume output is human-generated. Instead, without clear markers, we will increase our cynicism – devaluing and distrusting every output we come across. It is therefore imperative that we begin to clearly label AI and human-generated content.
About the Author
Nicholas (Nick) Barrow is a Research Associate at the Institute for Ethics in Technology. He’s primarily a Moral Philosopher, specialising in the Value of Consciousness and its intersection with both the Philosophy of Technology and the Philosophy of Well-Being. He most recently worked with Patrick Haggard at UCL on the Ethics of Haptic Technology and, before that, with Tania Duarte and Kanta Dihal on the We and AI and University of Cambridge research project Better Images of AI. As Artificial Intelligence Scholar, he achieved his masters in the Philosophy of AI from the University of York (supervised by Prof. Annette Zimmermann), having previously completed his first-class undergraduate degree in Philosophy from the University of Kent. To read more about his research, see his website.
References
Moffat, D. and Kelly, M., 2006. An investigation into people’s bias against computational creativity in music composition. Assessment, 13 (11)
Chamberlain, R., Mullin, C., Scheerlinck, B. and Wagemans, J., 2018. Putting the art in artificial: Aesthetic responses to computer-generated art. Psychology of Aesthetics, Creativity, and the Arts, 12 (2)
Lima, G., Zhunis, A., Manovich, L. and Cha, M., 2021. On the social-relational moral standing of AI: An Empirical study using AI-generated art. Frontiers in Robotics and AI, 8
This blog and its content are protected under the Creative Commons license and may be used, adapted, or copied without permission of its creator so long as appropriate credit to the creator is given and an indication of any changes made is stated. The blog and its content cannot be used for commercial purposes.